If you’re a SaaS founder trying to scale inbound without setting money on fire through paid ads, this is your edge. Because your buyers aren’t searching like they used to, and search engine optimization isn’t what it used to be.
If you’re still relying on keyword tools and volume metrics from five years ago, you’re flying blind while your competitors are using satellite data.
Today’s buyers don’t move in a straight line. They bounce from blog to G2, from demo video to the pricing page.
Machine learning SEO algorithms are how you keep up. They don’t track what users search for; they track what they do. What they click. What they ignore. What holds their attention.
Patterns, not guesses.
That’s why your strategy has to anticipate behavior, not react to it.
AI search optimization helps you show up where your buyers are deciding. And here’s how you can use that to outpace the pack.
AI isn’t Just Faster. It’s Smarter Than You Think.
AI no longer stops at keyword analysis - that era is over. It digs deeper: into behavior, patterns, and what happens after the click. It doesn’t track data. It learns from it.
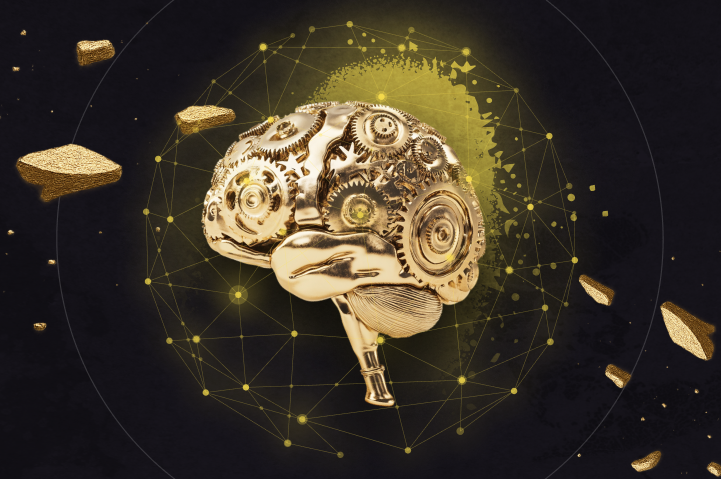
Think: How Google decides which onboarding tool comparison post to show your potential customers before they even know what to search for next?
Built on data from millions of searches, machine learning models can:
- Spot what’s rising (before tools show volume)
- Analyze how you interact with the content (clicks, scrolls, bounce rates)
- Detect subtle changes in how you phrase queries
- Cluster different queries by a shared intent
- Predict your next click by tracking every scroll, search, and signal (think: YouTube recommendations, Google suggestions)
If you know what machine learning is doing behind the curtain, then you know what to look for in the first place: AI search optimization.
Catching Early Signals Others Miss
Artificial intelligence can identify trends before the market knows they’re hot.
Think of it as your 24/7 SEO scout, scanning the horizon while others are still looking at last month's map.
Before your competitors publish a listicle on “top CRM integrations,” AI will tell you that buyers are already searching for “post-demo onboarding flows.” That’s your window.
ML models are trained to spot:
- Unusual increases in long-tail queries across forums, Reddit threads, or niche communities
- Topic pairings that signal new user needs (“sleep tracking” + “burnout recovery”)
- Rising chatter around emerging themes, even without a clear volume
These micro-signals suggest something’s brewing, and you can optimize your content before the surge hits.
The Actionable Insights Start After the SERP
SaaS buyers don’t just read. They compare, skim, bounce, or loop back later. Machine learning algorithms track:
- Did the user scroll past the intro?
- Did they bounce after 8 seconds?
- Did they click on a related internal link or head back to Google?
This helps models learn which content formats, structures, and topics satisfy user intent.
For your SEO efforts, this means:
- Creating content to maximize dwell time, not word count
- Using internal links to move users from one question to the next logical step
- Optimizing layout, not only text
Online visibility gets you in the door but relevance keeps you there.
And what you do next is what converts.
When Queries Shift, So Should Your Strategy
Search habits evolve. ML models can track when people start searching differently for the same thing. AI picks up on these shifts by comparing new query patterns to old ones.
Examples:
- “Email marketing software” → “Best way to automate follow-ups after demo requests”
- “CRM tools” → “Customer journey automation platforms”
This helps you:
- Align with how your target audience speaks today
- Detect the maturity level of a searcher (beginner vs expert)
- Adjust the tone, format, and even CTA positioning
Mapping Intent Across Fragmented Search Behavior
People use different words and terms for the same goal. Machine learning models group those terms not by exact match but by common outcomes.
Example:
- “Best CRM for startups”
- “Affordable sales tracking software for small teams”
- “HubSpot vs Pipedrive for early-stage companies”
Different phrasing, same intent. AI sees this and groups the intent, so instead of creating fragmented articles, you should:
- Build unified content hubs
- Optimize for broader SERP ownership
- Organize content to simplify decisions, not to overwhelm them.
Content Optimization That Anticipates the Next Click
This is where AI gets scary smart.
By analyzing millions of behavior patterns, models can predict what someone is likely to do next:
- What they will search for after a blog post
- What product will they consider after a case study
- What format do they prefer (video, long-form, checklist)
You see this in action with:
- Google’s “People also ask” or autocomplete
- Netflix recommendations
- Amazon’s “Frequently bought together”
For machine learning in SEO and AI content optimization strategies, this means you can:
- Preemptively build content for the next question
- Structure pages to guide the next action
- Design journeys, not answers
The Evolution of Search Behavior Analysis
Google doesn’t rank keywords.
It ranks answers.
That’s why AI helps you shift from optimizing for “best CRM for startups” to understanding what the searcher wants:
- Ease of setup?
- Feature comparisons?
- Budget-friendly options without sacrificing integrations?
Keywords are surface-level. They don’t explain why the person is searching, what problem they’re trying to solve, or how close they are to taking action.
Once you map the intent, you can:
- Serve the right CTA at the right time
- Guide them from curiosity to conversion
- Create less content, but get more results
Machine learning gives you proof, not opinions. It shows you what real users are doing, not what you think they’re searching for.
The Rise of Conversational and Question-Based Searches
Queries now sound like:
“What’s the easiest CRM to set up for a remote sales team with no tech support?”
AI-powered NLP tools can parse these complex queries and identify patterns that traditional tools miss.
That means they can:
- Break down complex, multi-part questions
- Understand intent, sentiment, and context
- Analyze the relationships between terms, topics, and the outcomes users care about
- Cluster related queries, even if they look nothing alike on the surface
Why This Beats Traditional Keyword Tools
Traditional SEO tools:
- Rely on exact-match or broad-match phrases
- Focus on volume, CPC, and competition metrics
- Often miss the nuance in how users phrase modern search queries (especially voice or mobile search)
While ML tools:
- Surface pain-driven queries like “why users drop off after day one” (signal deeper intent)
- Cluster related long-tail questions around user problems
- Reveal question structure and phrasing from actual search behavior (especially useful for building journey-based content)
Tools That Help:
- Frase.io: Takes user-intent signals and turns them into strategic content creation plans aligned with user behavior and what ranks.
- Surfer SEO & Clearscope: Use NLP to suggest semantically relevant terms from top-ranking content (great for depth, not for behavioral insights).
- AlsoAsked & AnswerThePublic: Visualize how users ask questions, helping you structure content around how people think and not just what they type.
Most of these tools integrate with:
- Google Search Console
- CMS platforms like WordPress or Webflow
- Analytics tools for performance tracking
These AI tools don’t just optimize - they’re reshaping how digital marketing teams approach content strategy, from blog planning to real-time user intent tracking.
Once you use them, you stop playing the “keyword match” game and start creating intent-aligned, high-value content.
How Machine Learning Models Interpret Search Behavior Differently
ML-powered systems analyze:
- User engagement patterns
- Bounce rate vs. dwell time
- Clickstream data across platforms
That learning actually comes from:
- Clicks
- Time on page
- Scroll depth
- Interaction with media (videos, CTAs, etc.)
These behaviors help algorithms refine rankings over time. If users keep clicking on result #3 and ignoring result #1, Google pays attention.
- The bounce rate shows when a user leaves a page without taking further action
- The dwell time tracks how long a user stays on your page before heading back to search results
Together, these are user satisfaction proxies. If someone stays 3 minutes and doesn’t bounce, the content probably met their needs. These signals help Google rank intent-matching pages higher, even if they’re not keyword-heavy.
Search engine algorithms use clickstream data, which is the path that users take across websites, search engine results, and platforms. This includes:
- What links users click on from the SERP
- How they move between related queries
- How they explore different content types (videos, forums, articles)
However:
- Google has the most powerful clickstream visibility (via Chrome, Android, YouTube, etc.).
- The majority of third-party tools estimate engagement patterns based on limited, surface-level input data.
So while ML models can learn from this behavior, not all tools can tap into it at the same level.
This is Where Most Marketers Fall Behind
Most digital marketers react.
While many still chase keyword rankings manually, leading SEO professionals are shifting toward behavior-first frameworks powered by machine learning and NLP.
The marketers who lead? They treat SEO like data science - analyzing scrolls, clicks, and drop-offs like behavioral signals, not just bounce stats.
AI tools give you:
- First-mover insight: This lets you optimize content before competitors realize there's demand.
- Semantic depth: Create relevant content that resonates with intent, not keywords (ChatGPT, Gemini, etc.)
Most of them (especially those using natural language processing and semantic analysis) can:
- Understand the why behind a query (informational vs commercial vs transactional intent)
- Include semantically related terms and concepts (not variations of the keyword)
- Build content that mirrors how humans think about the topic (better aligned with user expectations)
You can map the entire journey by analyzing:
- Sequential search behavior (what queries brought people to your page, what pages they visited next, and which pages had the highest drop-off or continuation rates)
- Content consumption across platforms (e.g., TikTok → Google → YouTube → product page)
- Multi-touchpoint intent (awareness → evaluation → conversion)
This allows you to design:
- Smarter content funnels
- Internal linking strategies that match the decision flow
- CTAs that reflect where the reader is in the journey
And when you act on these insights? Instead of guessing what comes next, you guide it.
How Machine Learning Algorithms Find SEO Gold
Google uses entity-based indexing (think: concepts, not keywords).
AI tools can mimic this by mapping:
- Entities (people, places, products)
- Their relationships (Apple → iPhone → Face ID → Privacy)
- Search context (tech vs fruit)
Intent Beyond Traditional Keyword Grouping
AI doesn’t group by match; it groups by meaning and intent.
A traditional tool might separate “churn reduction” from “product onboarding tips” or “customer success email templates.” But an AI model sees the full journey - a SaaS company trying to retain users and increase lifetime value.
That lets you build content hubs that rank across:
- Broad queries (e.g., “how to reduce SaaS churn”)
- Long-tail searches (e.g., “onboarding emails that improve feature adoption”)
- Adjacent opportunities (e.g., “CS playbooks for upselling during QBRs”)
Now you’re not only optimizing for a few scattered keywords; you’re capturing the full retention narrative that your buyers are actively navigating.
Someone's Blind Spots are Your Ranking Opportunities
Traditionally, content gaps meant chasing competitor rankings one keyword at a time.
Useful? Sometimes.
Effective? Rarely.
Because it leads to more of the same.
Modern AI flips that thinking. It doesn’t look at rankings, it looks at opportunity.
AI tools identify:
- High-interest queries with low-quality results
- Questions users ask that no one answers well
- Subtopics within a cluster that lack coverage
In short: where you can win. Fast.
Content gap analysis isn't about checking boxes anymore. It's about identifying unclaimed search trends.
Analyze competitors by:
- What they’re missing in their content themes
- What queries they’re not addressing fully
- Where their bounce or exit rates spike
Instead of guessing what to publish next, you:
- Build content that fills proven holes
- Show up where no one else is competing
- Win SERP real estate with less effort and more impact
That’s how you stop reacting to rankings - and start engineering them.
Target the Moments That Drive Decisions
People don’t search in a straight line. They search in spikes, triggered by curiosity, frustration, urgency, or the need to make a move.
Google calls these “I want to know/go/do/buy” moments.
Here’s what that looks like in practice:
- Day 1: “What is customer onboarding software?”
- Day 3: “Best SaaS onboarding tools for small teams”
- Day 5: “Userflow vs Appcues comparison”
That timeline tells you exactly:
- When they’re exploring
- When they’re evaluating
- When they’re ready to act
Your job? Align every blog, page, and help doc with the questions your users are already asking. That’s AI search optimization in action.
Machine Learning SEO: Implementation Strategies
Real-time insights from SEO machine learning tools help you catch slippage early, double down on what’s working, and respond faster than manual analysis allows.
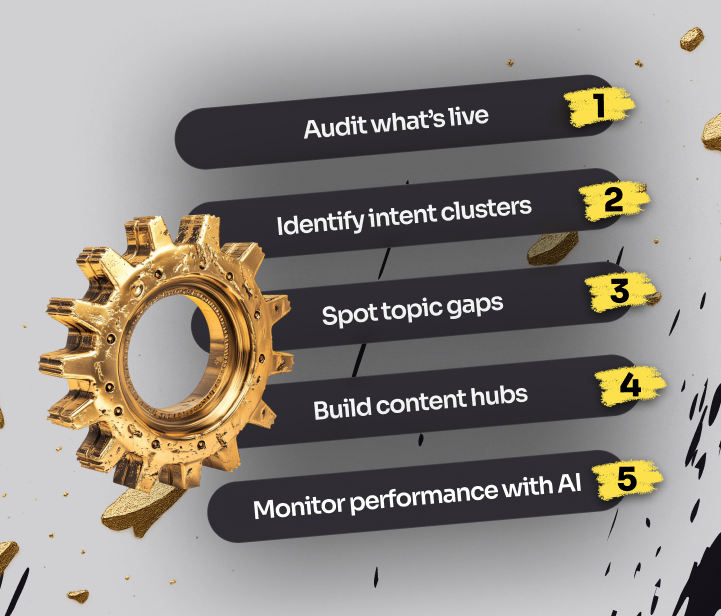
Step-by-Step Execution
- Audit what’s live
Strip back your current keyword-first SEO strategy. Find what ranks, what doesn’t, and what’s just filler.
Don’t just look for outdated posts - use machine learning to identify emerging trends that your competitors haven’t capitalized on yet.
- Identify intent clusters
Use AI tools (like Frase, ChatGPT, or AlsoAsked) to group queries by what the user wants, not what the tools say is popular.
- Spot topic gaps
AI models can flag where your content stops short… or never shows up.
It’s not about missing relevant keywords - it’s about missing entire conversations that those keywords are part of. That’s where ML models offer real strategic depth.
- Build content hubs
Create content by targeting core topics and interlink pages based on user flow, not internal wish lists.
- Monitor performance with AI
Track what’s working, what’s slipping, and what’s surfacing to optimize performance across your content funnel.
You can test variations of headlines, meta descriptions, and intro copy to see what drives engagement - and let AI recommend tweaks based on behavior signals.
Action Plan: Make AI Search Optimization Part of How You Operate
You need to be present when the intent is high and the stakes are real. Outranking competitors means nothing if your content isn’t showing up where decisions happen:
- On a mobile search during a sales call
- After a YouTube review, looking for proof
- On a “vs” page at 10 p.m., ready to convert
This is how it becomes part of your engine and not a side experiment:
- Audit your current marketing strategies.
- Pick platforms that surface patterns, not just keywords.
- Cluster content by user journey, not keyword volume.
- Don’t measure where you appear. Measure what users do once you’re there.
Before publishing new content, look for pages where you can optimize existing content to match today’s search behavior - often a faster win than starting from scratch.
Wrap Up
Forget replicating what already ranks. The real advantage is anticipating intent before it peaks. Understanding not only what people search for but why, when, and what they expect to find next.
Manual research can’t keep pace. Guesswork doesn’t scale.
And content without intent? Won’t land.
A lot of advice still clings to outdated takes on the SEO landscape - but what’s changed is how search engines interpret value, not just keywords.
AI search optimization is the only way to keep up.
Faster insights. Smarter structure. Real alignment with how buyers think, act, and convert.
Yes, search rankings matter. But ranking without relevance is a wasted effort.
If your SEO strategy still starts and ends with keyword research, you’re not just behind - you’re invisible where decisions are made.
You’re here to capture demand, guide decisions, and drive growth that compounds.
Frequently Asked Questions
Q: What is machine learning SEO and how is it changing search rankings?
A: Machine learning SEO uses AI-powered algorithms to analyze user behavior patterns - such as clicks, dwell time, and scroll depth - instead of just focusing on keyword matching. Search engines now prioritize content that aligns with user intent, anticipates next actions, and creates satisfying experiences, making behavior-first strategies essential for ranking.
Q: How does artificial intelligence impact SEO strategies?
A: Artificial intelligence impacts SEO by moving beyond static keyword targeting. It identifies emerging trends, understands searcher intent, and predicts behavior based on real-time interactions. Brands using AI-driven SEO tools can spot opportunities earlier, optimize for evolving queries, and create content hubs that mirror user decision journeys.
Q: Why are traditional keyword tools no longer enough for SEO success?
A: Traditional keyword tools focus on volume and CPC metrics, but they miss the nuance behind modern search queries. Today’s SEO requires understanding semantic relationships, user behavior signals, and question-based search patterns, which AI and machine learning models are built to capture and analyze at scale.
Q: How can machine learning help identify early SEO opportunities?
A: Machine learning models detect micro-signals, such as rising niche topics or new query combinations, long before volume shows up in keyword tools. This gives marketers a competitive advantage by allowing them to create high-relevance content before demand spikes and competitors flood the space.
Q: What role does user behavior analysis play in SEO today?
A: User behavior analysis is now central to SEO success. Search engines measure how users interact with content - tracking clicks, bounce rates, dwell times, and engagement paths - to determine if a page satisfies intent. Content that holds attention and guides logical next steps earns stronger rankings, regardless of keyword density.
Q: How can AI tools improve content strategy beyond keywords?
A: AI tools like Frase, ChatGPT, and Surfer SEO help marketers build journey-driven content by clustering queries based on shared user goals, not just keyword similarity. They reveal pain points, emerging trends, and content gaps, enabling brands to build smarter content hubs that dominate broader SERP territory.
Q: What is AI search optimization and why does it matter?
A: AI search optimization means designing SEO strategies that align with how machine learning models evaluate relevance, satisfaction, and user experience. It shifts focus from stuffing keywords to creating intent-aligned, high-value content that matches what users want - and what algorithms are rewarding.
Q: How does machine learning track and influence SEO rankings?
A: Machine learning tracks SEO rankings through behavior signals like click-through rates, session duration, scroll behavior, and content interactions. If users consistently engage more with a lower-ranked result, machine learning models adjust rankings over time, promoting content that better meets user expectations.
Q: How can marketers use AI to build better content hubs for SEO?
A: Marketers can use AI tools to cluster related queries by buyer journey stages, surface hidden user questions, and map out pillar-cluster strategies based on real search behavior. This approach builds authority across interconnected topics and ensures content is optimized for exploration, not just single queries.